Q* - Did OpenAI achieve AGI?
In Machine Learning, Q* is known as the convergence to the optimal action-value function. In OpenAI, this might have been the reason of the chaos around Sam Altman in the past weeks.
Letβs take a deep dive on why Q* might be playing a key role in AI really soon.
What is AGI?
Is there something after AGI?
Back to today: What is Q*?
Q-learning 101
Hands-on: Q-learning in Python
Simple example of Q-learning in Python
Q-learning: Practical application in the stock market
What is AGI?
AGI stands for Artificial General Intelligence, which refers to a type of artificial intelligence that has the ability to understand, learn, and apply knowledge in a way that is not specific to a particular task, domain, or narrow set of problems. It is an AI that has the capacity to reason, solve problems, plan, communicate, and integrate knowledge across a wide range of topics as effectively as, or more effectively than, a human being.
Here are some key characteristics often associated with AGI:
1. Learning and Reasoning: AGI can learn from experience and data, reason through problems, and apply logical processes to navigate new situations.
2. Transfer Learning: Unlike narrow AI, AGI can transfer learning from one domain to another. For example, an AGI that learns language could apply its understanding to learn programming.
3. Understanding Context: AGI can understand context and make judgments about how to behave appropriately in different situations, adapting to new environments with little to no additional input.
4. General Problem Solving: AGI is capable of problem-solving across a range of tasks that would typically require human intelligence, such as understanding natural language, recognizing objects, and emotional intelligence.
5. Autonomy: AGI systems can operate independently in a variety of environments and use reason to navigate and make decisions without human intervention.
6. Consciousness and Self-awareness: While still a subject of debate and research, some theories suggest that true AGI would possess some form of consciousness or self-awareness.
As of today, AGI is widely considered as a theoretical concept and has not been achieved. To get some context, current AI systems, including advanced machine learning models and neural networks, are considered narrow AI or ANI (Artificial Narrow Intelligence), meaning they are very good at performing specific tasks but cannot generalize their knowledge and skills to the wide array of tasks that a human can perform. The development of AGI is considered by many to be the holy grail of AI research, but it also raises important ethical, safety, and governance questions.
Is there something after AGI?
The next frontier after AGI is called Superintelligence, and it refers to an intellect that is much smarter than the best human brains in practically every field, including scientific creativity, general wisdom, and social skills. This concept is most often discussed in the realm of artificial intelligence and is considered an extension of the idea of Artificial General Intelligence (AGI).
The main aspects of superintelligence are:
1. Advanced Cognitive Abilities: A superintelligent AI would be able to outperform the brightest and most gifted human minds.
2. Problem-Solving Skills: Such an AI could solve problems that are currently intractable for humans, potentially finding solutions to complex scientific, mathematical, and social issues.
3. Learning and Adaptation: Superintelligence would have the ability to learn and adapt at an extraordinary pace, far exceeding human cognitive abilities.
4. Technological Mastery: It might have the capability to drive rapid technological growth, potentially leading to the creation of even more advanced forms of AI.
5. Autonomy: A superintelligent system could have the capacity to act autonomously with exceptional proficiency in multiple domains, possibly without human guidance or oversight.
The notion of superintelligence goes beyond existing advanced AI systems, which are designed to excel in specific tasks. The idea carries both optimistic and pessimistic views regarding the future:
- Optimists believe that superintelligence could help solve many of the world's problems, from curing diseases to halting climate change or even traveling to other stars.
- Pessimists worry about the potential risks, including ethical concerns and the possibility that a superintelligent AI could become uncontrollable or make decisions detrimental to human well-being.
Superintelligence is a topic of much speculation, ethical consideration, and philosophical debate, with prominent figures in science and technology discussing its potential impact on humanity. As of now, it remains a hypothetical scenario, with no existing AI systems reaching the levels of capability often described in superintelligence discussions.
Back to today: What is Q*?
In Machine Learning, Q* (pronounced Q-star) is known as the convergence to the optimal action-value function when implementing Q-learning in a Machine Learning model.
Q-learning 101
Q-learning is a type of reinforcement learning algorithm used in machine learning, a subset of artificial intelligence (AI). It's used to find an optimal action-selection policy for any given (finite) Markov decision process. Here's a breakdown of its key aspects:
1. Model-Free Approach: Q-learning is model-free, meaning it doesn't require a model of the environment. It's capable of learning solely from the experience of interacting with the environment.
2. Learning the Q-Value: The core of Q-learning is to learn the value of an action in a particular state. This value (Q-value) represents the expected utility of taking a given action in a given state and following a certain policy thereafter.
3. Bellman Equation: Q-learning uses the Bellman equation to update the Q-values. The equation calculates the expected future rewards that can be obtained from the next state. This allows the algorithm to make decisions that maximize the long-term reward.
4. Exploration vs. Exploitation: In Q-learning, there's a trade-off between exploration (trying new actions to discover their rewards) and exploitation (using actions known to yield high rewards). Balancing these two is crucial for effective learning.
5. Convergence: Under certain conditions, such as having a finite number of states and actions, and with adequate exploration, Q-learning is proven to converge to the optimal action-value function.
6. Applications: Q-learning is widely used in areas such as robotics, automatic control, economics, and gaming. It's particularly effective in situations where the environment is stochastic (randomly determined) and the full model of the environment is unknown.
In practice, Q-learning is implemented using a Q-table or a Q-network (in Deep Q-Learning, which integrates neural networks), where the rows represent the states, the columns represent the actions, and the values represent the expected rewards of taking an action in a state. The algorithm iteratively updates these values as it learns from new experiences.
Hands-on: Q-learning in Python
Simple example of Q-learning in Python
Let's create a simple example of Q-learning in Python. We'll use a very basic environment: a 1D world where the agent must move to the right end to win. This example is simplified to illustrate the concepts clearly.
The environment:
A linear world with 5 states (positions) labeled 0 to 4.
The agent starts at position 0.
The goal is to reach position 4.
Actions are moving left (0) or right (1).
A reward of 100 is given for reaching the goal, and a small negative reward (-1) for each step, to encourage efficiency.
Explanation of the Code:
Initialization: We initialize the environment parameters and the Q-table, which holds the Q-values for each state-action pair.
Action Selection: The choose_action function selects an action for the current state. It uses Ξ΅-greedy policy: with probability Ξ΅, it explores by choosing a random action, and with probability 1-Ξ΅, it exploits the best known action.
Learning: The update function updates the Q-values in the table using the Bellman equation. It takes the current state, next state, reward, and action to adjust the Q-value towards the expected long-term return.
Training Loop: We run multiple episodes where the agent interacts with the environment. In each episode, it starts from the initial state and makes decisions based on its Q-table, updating the table with each step.
Result: After training, the Q-table represents the learned policy, indicating the best action to take in each state.
When you run this code, you'll see the trained Q-table, where each row corresponds to a state and each column to an action. The values represent the expected long-term return for taking that action in that state, guiding the agent to the goal.
Q-learning: Practical application in the stock market
Let's create a more elaborate Q-learning example using an economic scenario. Imagine we have a simplified stock market environment where an agent decides whether to buy, hold, or sell stocks based on their prices.
Scenario Description:
States: The states are a combination of the agent's current portfolio status (e.g., amount of stock owned) and a simplified representation of the market condition (e.g., low, medium, or high prices).
Actions: The agent can choose to buy, hold, or sell stocks.
Rewards: The reward is based on the profit or loss made by the agent's actions. For example, selling at a higher price than the buying price yields a positive reward.
Objective: The agent's goal is to maximize profit over a series of trading days.
*The market conditions and stock prices will be simplified for the sake of the example.
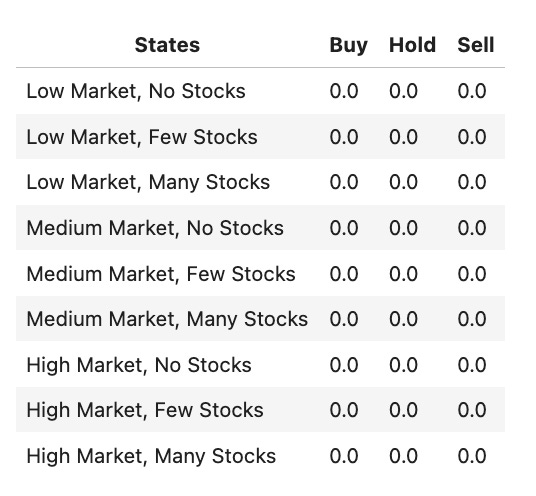
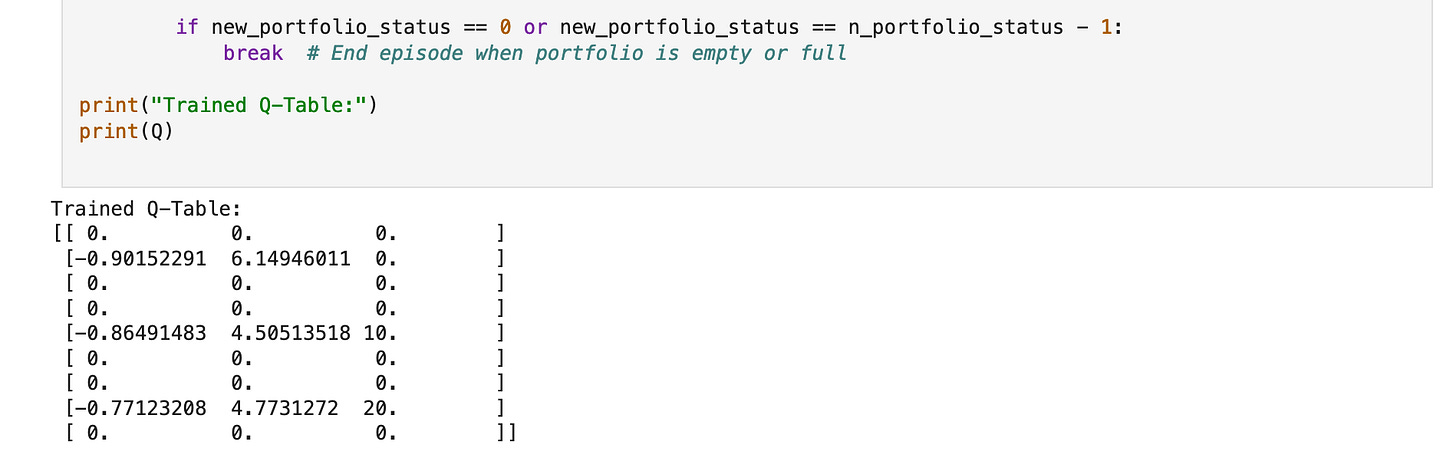
Remember: The Q-table in the output of the Q-learning algorithm represents the learned values (Q-values) for each combination of state and action.